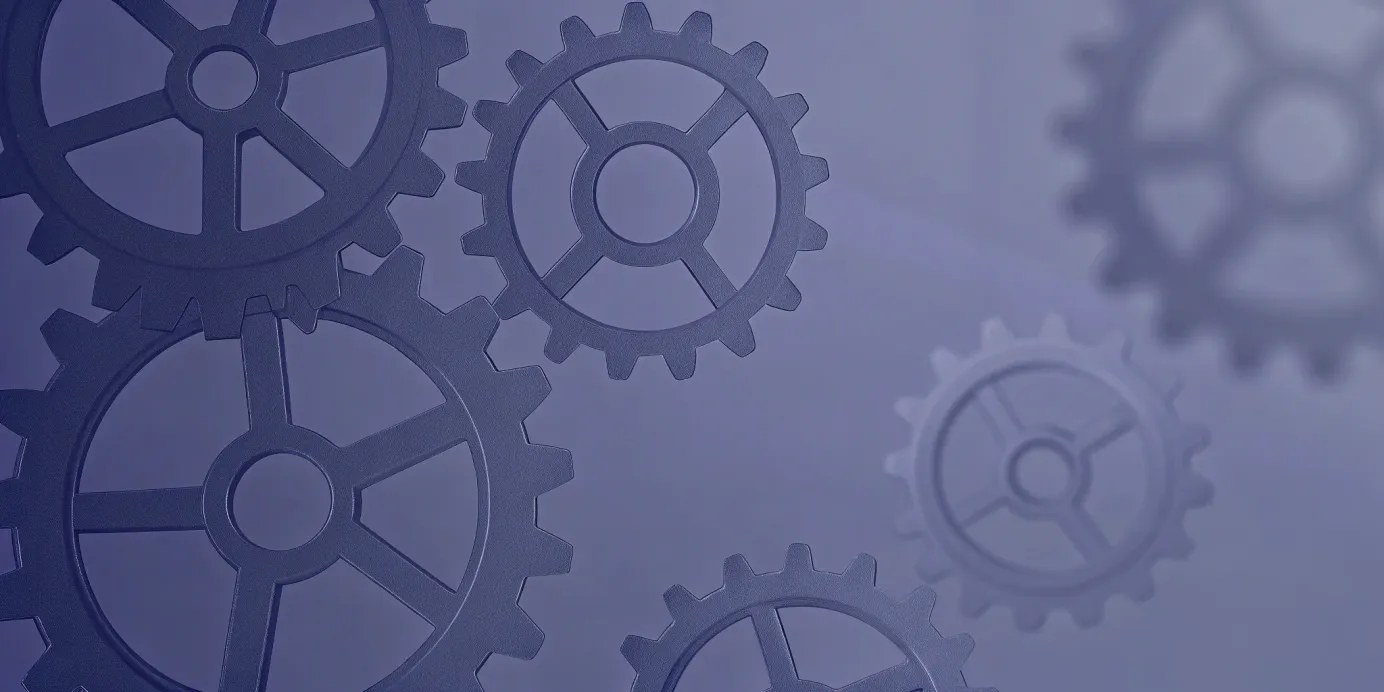
Kubernetes Pod Tolerations for Node Scheduling
Master pod tolerations in Kubernetes to control node scheduling effectively. Learn how to use tolerations to optimize your deployments and manage node resources.
Table of Contents
Need to schedule your Kubernetes pods onto the right nodes, especially those with specialized hardware like GPUs? Kubernetes tolerations give you fine-grained control over pod placement on tainted nodes. This post breaks down how tolerations work, the different types, and practical use cases to optimize your Kubernetes deployments.
This post explores how Kubernetes tolerations work, their different types and effects, and best practices for using them effectively. We'll cover practical examples and common use cases to help you master this essential aspect of Kubernetes scheduling. By the end of this post, you'll be able to use Kubernetes tolerations confidently to optimize your deployments and ensure your applications run smoothly.
Unified Cloud Orchestration for Kubernetes
Manage Kubernetes at scale through a single, enterprise-ready platform.
Key Takeaways
- Control pod scheduling with tolerations. Matching pod tolerations to node taints allows you to schedule pods onto nodes they would otherwise be unable to use. Use the key, value, effect, and operator fields for precise control.
- Understand the different toleration effects. NoSchedule prevents new pod scheduling, PreferNoSchedule discourages it, and NoExecute evicts existing pods. Select the effect that best suits your specific use case, such as reserving resources or handling node failures.
- Use tolerations strategically and monitor their impact. Overuse can complicate debugging. Document your tolerations and observe their effects on pod placement and resource utilization to ensure your cluster runs smoothly.
Understanding Kubernetes Pods, Nodes, and Taints
What are Kubernetes Pods?
The smallest deployable units
In Kubernetes, Pods are the smallest deployable units, representing a group of one or more containers deployed together on the same node. These containers within a Pod share resources like storage volumes, network namespaces, and an IP address, facilitating efficient communication and data sharing.
Containerization within Pods
While a Pod can contain multiple containers, the most common scenario is a single container per Pod. This design promotes modularity and separation of concerns. If your application requires multiple components, deploy them as separate Pods that communicate with each other, rather than combining everything into a single Pod. This simplifies management, scaling, and troubleshooting.
Ephemeral Nature and Lifecycle Management
Kubernetes Pods are ephemeral, designed to be created and destroyed dynamically based on application needs and cluster state. The Kubernetes control plane manages this lifecycle, ensuring the desired number of Pod replicas are always running. If a Pod fails, Kubernetes automatically creates a replacement, contributing to resilience and high availability.
What are Kubernetes Nodes?
The worker machines
Nodes are the worker machines—physical servers or virtual machines—in a Kubernetes cluster. Each node, managed by the Kubernetes control plane, runs Pods. The control plane schedules Pods onto available nodes based on resource requirements and other constraints.
Resource management on nodes
Each node has finite resources (CPU, memory, and storage). The Kubernetes scheduler considers these constraints when placing Pods, preventing resource starvation and ensuring application stability. Resource Requests and Limits provide fine-grained control over resource distribution among your Pods.
Introduction to Taints
Purpose and functionality of taints
Taints are "keep-off" signals applied to Kubernetes nodes, repelling specific Pods from being scheduled. They mark a node as unsuitable for certain workloads. For example, taint a node with specialized hardware (like a GPU) to ensure only GPU-requiring Pods are scheduled there, optimizing resource utilization.
How taints restrict pod scheduling
Taints have three effects: `NoSchedule`, `PreferNoSchedule`, and `NoExecute`. `NoSchedule` prevents new Pods without a matching toleration from being scheduled. `PreferNoSchedule` discourages scheduling but doesn't prohibit it. `NoExecute` evicts existing Pods without a matching toleration, offering granular control over pod placement based on hardware requirements, node roles, or maintenance.
Deep Dive into Kubernetes Tolerations
Tolerations override taints' "keep-off" signals, enabling Pods to be scheduled onto tainted nodes. A Pod needs a toleration matching the node's taint to be scheduled there. This offers flexibility in pod placement, ensuring Pods land on appropriate nodes. While tolerations allow scheduling, they don't guarantee it; the scheduler also considers resource availability and other constraints.
What are Kubernetes Tolerations?
Definition and Purpose
In Kubernetes, toleration allows a pod to run on nodes with matching taints. Without the right toleration, the pod can't be scheduled on tainted nodes. This control is essential for managing where pods run, particularly when you have specialized hardware or nodes with specific conditions. Tolerations give certain pods permission to use resources that other pods can't, ensuring your workloads run where they should.
How Tolerations Work with Taints
Taints and tolerations function like a lock and key. Taints, applied to nodes, mark them as unsuitable for pods without matching tolerations. A taint has a key
(like gpu), a value
(like true
), and an effect. The effect determines how the taint restricts pod scheduling. The Kubernetes scheduler checks a node's taints against a pod's tolerations. If a pod lacks a matching toleration for any taint on a node, the pod is affected according to the taint's effect.
For example, a NoSchedule effect prevents pods without a matching toleration from being scheduled on that node. This system lets you reserve nodes for specific workloads, ensuring resource-intensive applications have access to the necessary hardware.
Taint and Toleration Matching Logic
Key-Value Matching
Taints and tolerations work together based on matching keys and values. A toleration in a pod specification must include a key
field that corresponds to the taint's key on a node. If the taint also specifies a value
, the toleration must have a matching value
as well. Think of it like a lock and key system: only the correct key (toleration) can unlock (allow scheduling on) a node with a specific lock (taint). For more details, refer to the Kubernetes documentation on taints and tolerations.
Tolerations also have an operator
field that determines how the key-value matching works. If the operator is Equal
, the toleration's key and value must match the taint's key and value exactly. If the operator is Exists
, only the key needs to match; the value is ignored. This flexibility allows for broader matching when specific values aren't critical.
Effect Matching
The effect
field of a taint specifies how it restricts pod scheduling. There are three possible effects: NoSchedule
, PreferNoSchedule
, and NoExecute
. NoSchedule
prevents new pods from being scheduled on the tainted node. PreferNoSchedule
indicates a preference against scheduling pods on the node, but doesn't strictly prohibit it. The scheduler will try to find other suitable nodes, but if none are available, it may still schedule the pod on the tainted node. A good overview of these effects can be found in this Densify blog post.
NoExecute
is the most restrictive effect. It not only prevents new pods from being scheduled but also evicts any existing pods that don't have a matching toleration. Understanding these effects is crucial for controlling pod placement based on node conditions.
Multiple Taints and Tolerations
Nodes can have multiple taints, and pods can have multiple tolerations. The Kubernetes scheduler evaluates these as a series of filters. For a pod to be scheduled on a node, it must have a matching toleration for every taint on the node with a NoSchedule
or NoExecute
effect.
If even one of these taints is unmatched, the pod won't be scheduled or will be evicted, respectively. This allows for complex scheduling scenarios where multiple conditions must be met. For example, a node might have taints for specific hardware (GPU) and an operating system version. Only pods with tolerations for both taints would be allowed to run on that node. This granular control ensures that pods are deployed only on nodes that meet their specific requirements. The Kubernetes documentation provides further details on how multiple taints and tolerations interact.
Kubernetes Toleration Types and Effects
Tolerations control how pods interact with taints. Understanding the different types and effects of tolerations is crucial for managing your Kubernetes workloads effectively. Each toleration corresponds to a specific taint effect, allowing fine-grained control over pod scheduling and execution.
NoSchedule Effect
The NoSchedule
effect prevents new pods from being scheduled onto tainted nodes. Think of it as a soft restriction. If a node carries a taint with the NoSchedule
effect, only pods with corresponding toleration can be placed there. Existing pods on the node remain unaffected. This is useful when you want to dedicate certain nodes for specific workloads without disrupting currently running pods.
For example, you might reserve nodes with GPUs for machine learning (ML) jobs by tainting them and adding matching tolerations to your machine learning pods. This allows you to manage specialized hardware effectively within your cluster.
PreferNoSchedule Effect
The PreferNoSchedule
effect acts as a gentle nudge to the scheduler. The scheduler tries to avoid placing new pods on nodes with this taint, but it's not a hard rule. If other suitable nodes aren't available, the scheduler might still schedule pods on the tainted node. This is helpful when you want to prioritize certain workloads on specific nodes without strictly enforcing their placement. For instance, you could use this to steer batch processing jobs towards a set of nodes while still allowing other workloads to run there if necessary.
NoExecute Effect
The NoExecute
effect is the strictest of the three. It not only prevents new pods from being scheduled on the tainted node but also evicts any existing pods that don't have a matching toleration. This is essential when you need to guarantee that only specific pods can run on a node, perhaps for security or resource isolation reasons. A practical example is isolating nodes for handling sensitive data. By tainting these nodes with the NoExecute
effect and applying the correct tolerations only to authorized pods, you ensure that no other workloads can access those nodes. This approach strengthens your cluster's security posture.
Automatic Taints and Tolerations in Kubernetes
Kubernetes isn't just about manually applying taints and tolerations. It automates these processes to handle common node problems and ensure your applications remain resilient. This automation simplifies cluster management and improves the reliability of your deployments. For a deeper dive into these concepts, explore the Kubernetes documentation on taints and tolerations.
Automatic Tainting for Node Problems
Kubernetes automatically taints nodes experiencing problems. For instance, if a node is under memory pressure, has network issues, or becomes unreachable, Kubernetes applies taints to signal these conditions. This prevents new pods from being scheduled onto the affected node, giving it time to recover. This automated response helps maintain the stability of your cluster by isolating problematic nodes. For more details, refer to the Kubernetes documentation.
Default Tolerations for Common Taints
To avoid disrupting essential system services, Kubernetes provides default tolerations for common taints like node.kubernetes.io/not-ready
and node.kubernetes.io/unreachable
. Most pods inherit these default tolerations, allowing them to continue running for a short period (usually five minutes) even if a node becomes temporarily unavailable. DaemonSet pods, crucial for cluster maintenance, have a special default toleration for these taints with no time limit, ensuring they can always run on nodes, even during recovery. The official documentation provides further information on these default tolerations.
The Unschedulable Taint
The node.kubernetes.io/unschedulable
taint marks a node as completely unavailable for scheduling. Kubernetes uses this taint when a node is being drained, shut down, or otherwise taken out of service. Unlike other taints, unschedulable
affects all pods, including those with default tolerations. This ensures that no new pods are scheduled on the node while it's being maintained or decommissioned. You can manually add this taint, but Kubernetes also applies it automatically during certain operations, such as cluster upgrades or node maintenance. Learn more about managing unschedulable nodes in this Densify guide.
Implement Tolerations in Kubernetes Deployments
This section covers how to put tolerations into practice within your deployments.
Configure Tolerations with YAML
You configure tolerations directly within your pod specifications using YAML. Here's a basic example:
apiVersion: v1
kind: Pod
metadata:
name: ml-pod
spec:
tolerations:
- key: "dedicated-node"
operator: "Equal"
value: "gpu-worker"
effect: "NoSchedule"
In this snippet, the tolerations field contains a list of individual toleration settings. This specific configuration allows the pod to be scheduled on a node tainted with key: "dedicated-node"
, value: "gpu-worker"
, and effect: "NoSchedule"
. Without this toleration, the pod wouldn't be allowed on such a node.
Apply Tolerations to Pods
It's important to understand that tolerations are applied at the pod level. This means the toleration configuration needs to reside within the pod's specification. This gives you granular control over which pods can tolerate which taints. A pod with toleration effectively says, "I can handle this taint, even if other pods can't." This mechanism is crucial for managing workloads with varying requirements.
Advanced Configuration: Operator and Value Fields
The key, operator, value, and effect fields offer fine- control over how tolerations work. Let's break down the fields:
- key: This field identifies the specific taint the toleration addresses. It must match the key of the taint.
- operator: This field defines how the value of the toleration is compared to the value of the taint. You have two options:
- Equal: The value in the toleration must exactly match the value in the taint. This is the most common scenario.
- Exists: The presence of the key in the taint is sufficient. The value is ignored. This is useful when you only care about the existence of a taint, not its specific value.
- value: This field specifies the value to be compared against the taint's value when the operator is set to Equal.
- effect: This field must match the effect of the taint. The effects determine how the taint impacts pod scheduling.
By understanding these fields, you can create precise tolerations that match your specific scheduling needs.
Using kubectl for Taint and Toleration Management
Adding and Removing Taints
You can manage taints directly with kubectl
. This allows for dynamic adjustments and fine-grained control over your node configurations. To add a taint to a node, use the following command structure:
kubectl taint nodes <node name> <taint key>=<taint value>:<taint effect>
For example, to add a taint indicating a node has GPUs, you might use:
kubectl taint nodes worker-1 gpu=present:NoSchedule
This command taints worker-1
, preventing pods without a matching toleration from being scheduled. Removing a taint is equally straightforward:
kubectl taint nodes <node name> <taint key>:<taint effect>-
So, to remove the GPU taint from worker-1
, you'd use:
kubectl taint nodes worker-1 gpu:NoSchedule-
This dynamic control is essential for adapting to changing workload needs and ensuring efficient resource utilization. For more details, refer to the Kubernetes documentation on taints and tolerations.
Managing Namespaces and Deployments
Tolerations are applied at the pod level. This means they need to be specified within the pod's YAML configuration, typically as part of your deployment manifests. This granular approach allows you to control precisely which pods can tolerate which taints, ensuring that workloads are scheduled correctly across your cluster. When managing deployments across multiple namespaces, ensure that the tolerations within each pod's specification align with the taints present on the nodes in those namespaces. This targeted approach prevents scheduling conflicts and ensures your applications run smoothly. For complex deployments, consider using a tool like Plural to manage configurations and automate deployments across your cluster.
Inspecting Pods and Events
Monitoring pod status and related events is crucial for maintaining a healthy Kubernetes cluster. You can use kubectl
to inspect pods and identify any issues related to taints and tolerations. The kubectl describe pod <pod name>
command provides detailed information about a pod's status, including any events related to scheduling or eviction. Look for events with reasons like TaintTolerationMismatch
, which indicate that a pod couldn't be scheduled due to missing or mismatched tolerations.
For cluster-wide monitoring and observability best-practices, consider using tools like Prometheus. A query like kube_pod_status_reason{reason='Evicted'} > 0
can alert you to pod evictions, allowing you to investigate and address the underlying causes promptly. Combining kubectl
inspections with monitoring tools provides a comprehensive view of your cluster's health and helps you quickly identify and resolve any taint and toleration-related issues. For a deeper dive into troubleshooting Kubernetes, explore this guide on effective troubleshooting strategies.
Common Use Cases for Kubernetes Tolerations
Tolerations are a key part of managing how your pods get scheduled in a Kubernetes cluster. Here are some common scenarios where they're especially useful.
Manage Specialized Hardware Resources
You can use taints and tolerations to dedicate nodes with specialized hardware, such as GPUs or high-performance SSDs, to specific workloads. For example, if you have a set of nodes with GPUs and you want to ensure that only pods requiring GPUs get scheduled on them, you would taint these nodes and add a corresponding toleration to the pods that require GPUs. This prevents other pods that don't need these resources from consuming them.
Ensure High Availability
Kubernetes automatically adds tolerations for common node problems like node.kubernetes.io/not-ready
and node.kubernetes.io/unreachable
to your pods. These have a tolerationSeconds
value of 300, which means your pod will continue running on a node experiencing these issues for five minutes before being evicted. This built-in behavior provides a basic level of high availability, giving your application some time to handle transient node issues. You can adjust the tolerationSeconds value or add your own tolerations to fine-tune this behavior based on your application's specific needs.
Handle Node Failures Gracefully
Tolerations play a crucial role in handling node failures gracefully. When a node experiences problems like memory pressure or network issues, Kubernetes automatically taints the node. Pods without the corresponding tolerations are then evicted, allowing Kubernetes to reschedule them onto healthy nodes. This automated response helps prevent cascading failures and ensures that your applications remain available even when individual nodes fail. By strategically using tolerations, you can ensure that your applications can tolerate various node failures and maintain their desired state.
Dedicated Nodes for Specific Workloads
In Kubernetes, managing specialized hardware resources effectively is crucial for optimizing application performance. Taints and tolerations serve as powerful tools to ensure that only the appropriate workloads are scheduled on dedicated nodes. For instance, if you have nodes equipped with GPUs, you can taint these nodes to restrict pod access to only those pods that require GPU resources. This is achieved by applying a taint with a specific key and value, such as key: "dedicated-node"
and value: "gpu-worker"
, along with a NoSchedule
effect. Pods that need GPU access must then include a corresponding toleration in their specifications.
This approach not only prevents other pods from consuming these valuable resources but also ensures that resource-intensive applications have the necessary hardware to function optimally. By implementing this strategy, you can effectively manage your cluster's resources and enhance the performance of critical applications. For workloads that might not *require* specialized hardware but could benefit from it, using the PreferNoSchedule
toleration offers flexibility. This allows you to prioritize certain pods on these dedicated nodes while still permitting other workloads to run there if necessary, maximizing resource utilization.
Moreover, the flexibility of tolerations allows you to fine-tune pod scheduling based on your specific needs. For example, you might reserve nodes for machine learning jobs by tainting them and adding matching tolerations to your ML pods. This targeted approach helps manage specialized hardware effectively within your cluster, ensuring that workloads are allocated to the most suitable nodes. This granular control is especially valuable when working with Infrastructure as Code (IaC), where you can dynamically provision and configure nodes with specific hardware and then use taints and tolerations to orchestrate deployments precisely. For instance, with Plural, you can manage your Terraform configurations to deploy GPU-enabled nodes and then automatically taint them as part of your IaC workflow.
Best Practices for Using Kubernetes Tolerations
Working with taints and tolerations can be tricky. Following these best practices will help you avoid common pitfalls and ensure your deployments run smoothly.
Apply Taints and Tolerations Strategically
Taints and tolerations are powerful tools, but overuse can lead to scheduling complexity. Use them judiciously, focusing on specific use cases like dedicating nodes to particular workloads or handling hardware failures. Start by identifying the nodes you want to taint and the pods that need to tolerate those taints. Clearly define your strategy before implementation to avoid unintended consequences.
Implement Toleration Seconds
The tolerationSeconds
field provides granular control over pod eviction when using the NoExecute
effect. This field specifies how long a pod can remain on a tainted node after a matching taint is added. Without tolerationSeconds, pods with matching tolerations will remain on the node indefinitely, even if they shouldn't be there. By setting a value, you can ensure that pods are eventually evicted, allowing you to perform maintenance or reschedule pods to more appropriate nodes.
Automate Toleration Management
Manually adding tolerations to each pod definition can be tedious and error-prone, especially in large deployments. Automate this process using admission controllers to ensure consistency and reduce the risk of misconfigurations. Admission controllers intercept pod creation requests and can automatically add the necessary tolerations based on predefined rules.
Test and Deploy Gradually
Before applying taints and tolerations to your production cluster, thoroughly test your configuration in a non-production environment. Start with a small subset of nodes and pods to validate your strategy and identify any potential issues. Gradual rollout allows you to refine your approach and avoid widespread disruptions.
Understanding the Three Taint Effects
Taints influence how pods interact with nodes. Each taint has an effect that determines how it restricts pod scheduling. Understanding these three effects is crucial for managing your workloads:
- `NoSchedule`: This effect prevents new pods from being scheduled onto tainted nodes. Only pods with a corresponding toleration can be placed on a node with a `NoSchedule` taint. Existing pods on the node remain unaffected. This is useful for reserving resources for specific workloads, like dedicating certain nodes for your machine learning jobs.
- `PreferNoSchedule`: This effect acts as a soft preference for the scheduler. The scheduler will try to avoid placing new pods on nodes with this taint, but it’s not a strict rule. If other suitable nodes aren't available, the scheduler might still use the tainted node. This is helpful when you want to prioritize certain workloads—like batch processing jobs—on specific nodes without strictly enforcing their placement.
- `NoExecute`: This is the strictest taint effect. It prevents new pods from being scheduled on the tainted node and evicts any existing pods without a matching toleration. This guarantees that only specific pods can run on a node, which is useful for security or resource isolation. For example, you might use this to isolate nodes handling sensitive data. Learn more about taint effects.
Leveraging Custom Admission Controllers
Managing tolerations manually can become complex as your cluster scales. Automating this process with custom admission controllers is a best practice. These controllers intercept pod creation requests before they're applied to the cluster, allowing you to automatically add the necessary tolerations based on predefined rules. This ensures consistency and reduces misconfigurations. For example, an admission controller could automatically add a toleration for a "gpu" taint to any pod requesting a GPU resource, simplifying deployments and ensuring correct pod scheduling without manual intervention. Read more about automating toleration management.
Challenges and Pitfalls of Kubernetes Tolerations
While tolerations offer powerful control over pod scheduling, using them effectively requires understanding potential pitfalls. Misconfigured tolerations can lead to unexpected behavior and instability. Let's explore some common challenges and how to avoid them.
Understand Tolerations vs. Taints
Tolerations and taints work together but have distinct roles. Taints mark nodes with specific attributes, indicating that certain pods shouldn't be scheduled there. Tolerations, configured within pods, allow those pods to be scheduled on tainted nodes despite the taint. Think of taints as "keep out" signs and tolerations as exceptions to that rule. A pod without toleration for a specific taint won't be scheduled on a node with that taint.
Avoid Overusing Tolerations
It's tempting to use tolerations liberally, but overusing them can create complexity. Too many tolerations can make debugging scheduling issues difficult. If every pod tolerates every taint, you lose the benefit of targeted scheduling. Strive for the principle of least privilege: only apply tolerations when absolutely necessary. For example, if you have a pod that requires a GPU, use node selectors or affinity to target GPU-equipped nodes rather than tolerations. This keeps your configuration cleaner and more predictable. Overuse can also mask underlying infrastructure problems; if you're constantly adding tolerations to address scheduling issues, it might indicate a resource bottleneck or a misconfigured cluster.
Consider Node Conditions
Node conditions, like Ready
, OutOfDisk
, or MemoryPressure
, provide valuable insights into the state of your nodes. Before applying taints and tolerations, consider these conditions. For instance, if a node is experiencing memory pressure, adding a taint might exacerbate the issue by attracting more pods. Instead, address the underlying resource constraint. Similarly, during node maintenance, using the built-in node.kubernetes.io/maintenance
taint allows you to gracefully drain pods before taking the node offline. This ensures minimal disruption to your running applications.
Document Your Tolerations
Clear documentation is crucial for any Kubernetes configuration, and tolerations are no exception. Document which taints you're using, why they're necessary, and which pods tolerate them. This helps your team understand the scheduling logic and prevents accidental disruptions. Consider using annotations within your pod specifications to explain the purpose of each toleration. This makes your configuration self-documenting and easier to maintain. A well-documented approach to taints and tolerations simplifies troubleshooting and collaboration within your team.
Comparing Taints and Tolerations with Other Scheduling Methods
Kubernetes offers several methods for influencing pod scheduling. While taints and tolerations provide a powerful mechanism for controlling pod placement, understanding how they compare to other scheduling features like node affinity and pod anti-affinity is crucial for selecting the right tool for the job. Let's explore these differences.
Taints and Tolerations vs. Pod Anti-Affinity
Taints and tolerations work by repelling pods from specific nodes. A taint on a node effectively says, "Pods stay away unless you have a specific toleration." This is like a lock-and-key system, where the toleration is the key that unlocks access to the tainted node. Pod anti-affinity, on the other hand, focuses on preventing pods with matching labels from being co-located on the same node. Think of it as a force field that pushes similar pods apart. While both mechanisms influence pod placement, taints and tolerations operate at the node level, repelling pods, whereas pod anti-affinity works at the pod level, preventing co-location.
For example, if you want to ensure that two instances of a critical application don't run on the same node to prevent a single point of failure, pod anti-affinity is the appropriate choice. Conversely, if you have a node with specialized hardware and want to reserve it for specific pods, taints and tolerations are more suitable.
Taints and Tolerations vs. Node Affinity
Node affinity works by attracting pods to nodes with specific labels. It's a way of saying, "I prefer pods to run on nodes with these characteristics." This contrasts with taints and tolerations, which repel pods from nodes unless they have matching tolerations. Node affinity is about preference and attraction, while taints and tolerations are about restriction and permission. They serve different but complementary purposes.
Combining Taints and Node Affinity
While distinct, node affinity and taints and tolerations can be used together for powerful control over pod scheduling. You can use node affinity to attract pods to a group of nodes with specific labels, while simultaneously using taints to repel pods from a subset of those nodes that have additional, undesirable characteristics. This combined approach allows for nuanced scheduling decisions, ensuring that your pods land on the most appropriate nodes based on a combination of desired attributes and restrictions. For more on how Plural uses node affinity and taints, see our architecture page.
For instance, you might have a group of nodes labeled for a specific application tier. You can use node affinity to ensure that application pods are scheduled within that tier. Within that tier, you might have some nodes with older hardware. You can taint these nodes and add tolerations to only a subset of your application pods, allowing you to gradually phase out the older hardware without disrupting the entire application.
Troubleshoot and Debug Kubernetes Tolerations
Working with tolerations can be tricky. Let's break down common issues, debugging techniques, and how to monitor the effects of your tolerations.
Common Issues and Solutions
One common mistake is misconfigured tolerations. A typo in the key or effect can prevent pods from scheduling correctly. Double-check your YAML files and ensure the values align with your taints. Another frequent issue is overusing tolerations. Granting a pod overly broad tolerations can lead to unintended scheduling onto unsuitable nodes. Start with specific tolerations and broaden them only if necessary. Finally, remember that tolerations don't override node selectors or affinity. If a node doesn't meet a pod's other scheduling requirements, the toleration won't help. Review your pod's specifications to ensure all scheduling criteria are met. Simple configurations are best, especially when you're first starting out with Kubernetes. Overly complex setups can lead to errors and make debugging difficult.
Debugging Techniques
When troubleshooting toleration issues, start by examining pod descriptions and events using kubectl describe pod <pod-name>
. This command reveals scheduling decisions and any related errors. Next, inspect the nodes in your cluster with kubectl get nodes
and kubectl describe node <node-name>
to verify taints are correctly applied. If you're still stuck, check the control plane logs for more detailed information. Remember, taints and tolerations work together. A solid understanding of how they interact is crucial for effective debugging.
Monitor Toleration Effects
After applying tolerations, monitor their impact on your cluster. Observe pod placement using kubectl get pods -o wide
to ensure pods land on the intended nodes. Monitor resource utilization on nodes with taints to confirm workloads are balanced effectively. Metrics like CPU and memory usage can indicate whether tolerations are distributing pods as expected. If you see imbalances, adjust your tolerations or taints accordingly. By tracking pod placement and resource usage, you can ensure your tolerations are working as intended and contributing to overall cluster performance and reliability.
To streamline this process, consider using platforms like Plural. Plural offers a unified interface for managing your entire Kubernetes fleet. From resource monitoring to log viewing, its feature-rich dashboard simplifies Kubernetes operations, helping you maintain efficiency and balance across your cluster.
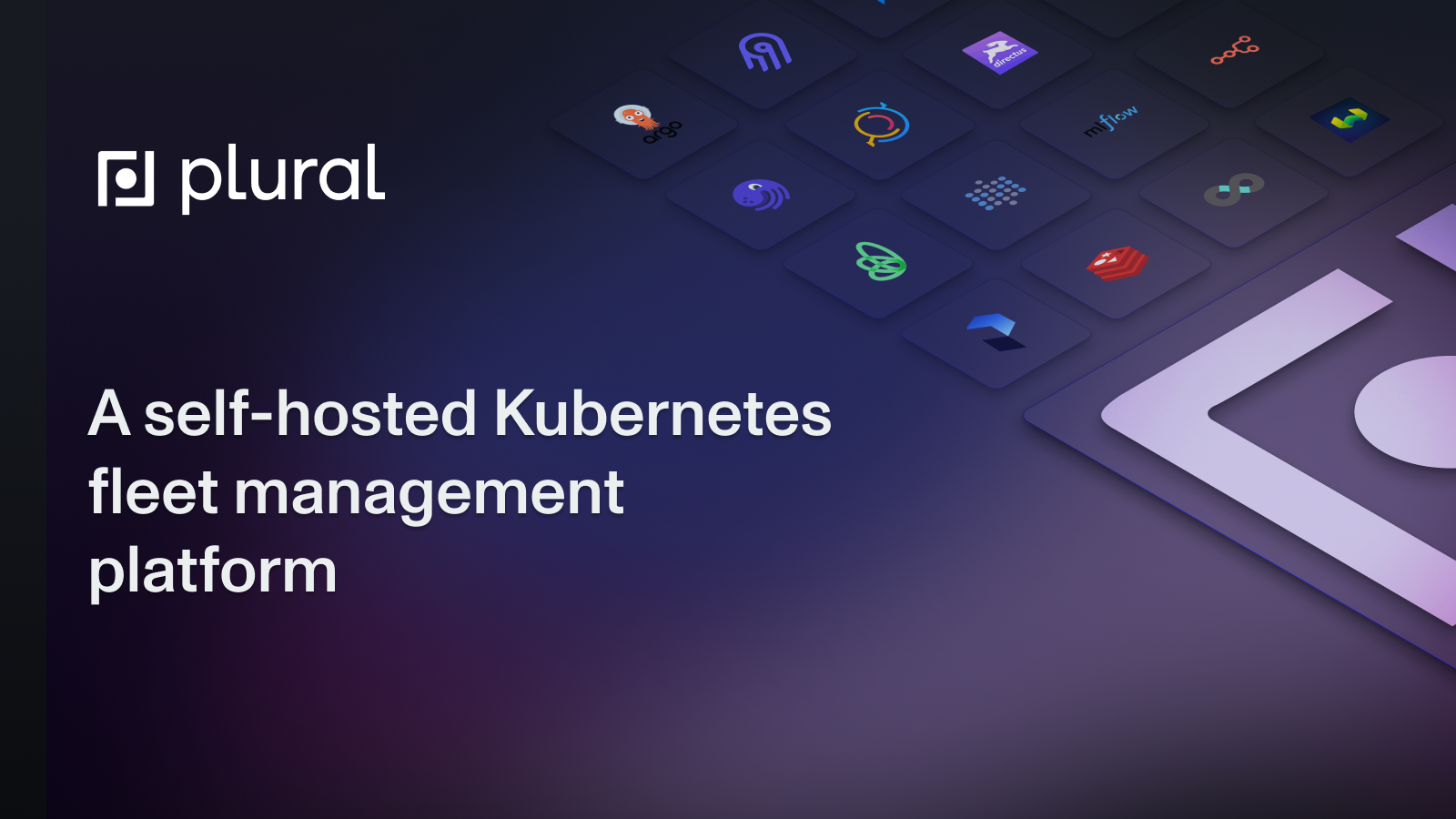
Related Articles
- The Quick and Dirty Guide to Kubernetes Terminology
- The Essential Guide to Monitoring Kubernetes
- Why Is Kubernetes Adoption So Hard?
- Understanding Deprecated Kubernetes APIs and Their Significance
- Kubernetes: Is it Worth the Investment for Your Organization?
Unified Cloud Orchestration for Kubernetes
Manage Kubernetes at scale through a single, enterprise-ready platform.
Frequently Asked Questions
How do tolerations interact with other pod scheduling constraints like node selectors and affinity?
Tolerations don't override node selectors or affinity rules. A pod must satisfy all its scheduling requirements to be placed on a node. Tolerations simply allow a pod to be considered for nodes it would otherwise be excluded from due to taints. If a node doesn't meet a pod's node selector or affinity requirements, the toleration won't have any effect. Think of it this way: tolerations address taints, while selectors and affinity address other placement criteria. They work in conjunction, not in opposition.
What's the difference between NoSchedule, PreferNoSchedule, and NoExecute toleration effects?
These effects determine how a taint restricts pod scheduling. NoSchedule
prevents new pods from being scheduled on a tainted node but doesn't affect existing pods. PreferNoSchedule
acts as a preference; the scheduler tries to avoid placing pods on tainted nodes but might do so if no other suitable nodes are available. NoExecute
is the strictest; it evicts existing pods and prevents new pods from being scheduled on the tainted node.
How can I automate the management of tolerations across my deployments?
Manually managing tolerations can be cumbersome. Kubernetes admission controllers offer a way to automate this process. They intercept pod creation requests and can automatically add the necessary tolerations based on predefined rules. This ensures consistency and reduces the risk of errors. You can also use templating tools like Helm to manage tolerations as part of your deployment definitions.
What are some common pitfalls to avoid when using tolerations?
Overusing tolerations can lead to scheduling complexity and make debugging difficult. Apply tolerations strategically, only when necessary. Another common mistake is misconfiguring the key or effect fields, which can prevent pods from being scheduled correctly. Always double-check your YAML. Finally, remember that tolerations don't override other scheduling constraints.
How can I troubleshoot toleration-related issues in my cluster?
Start by examining pod descriptions and events using kubectl describe pod <pod-name>
. This command provides insights into scheduling decisions and errors. Inspect your nodes with kubectl get nodes
and kubectl describe node <node-name>
to verify taints are correctly applied. If necessary, check the control plane logs for more detailed information. Tools like kubectl logs
can help pinpoint the source of scheduling problems.
Newsletter
Join the newsletter to receive the latest updates in your inbox.